In today’s digital era, businesses across the globe are embracing advanced technologies to enhance productivity, cut operational costs, and stay competitive. Among these technologies, machine learning (ML) has emerged as a game-changer. ML enables businesses to harness data for smarter decision-making, streamlined processes, and real-time automation. By leveraging artificial intelligence (AI), organizations can not only automate repetitive tasks but also gain actionable insights from their data—fostering innovation and long-term growth.
\Machine learning applications span a wide range of industries, from finance and healthcare to retail, logistics, and manufacturing. As algorithms evolve and data accessibility improves, ML is reshaping how businesses operate and interact with their customers. Whether it’s predictive analytics in supply chains or intelligent chatbots in customer service, ML-driven automation is no longer a luxury—it’s becoming a necessity.
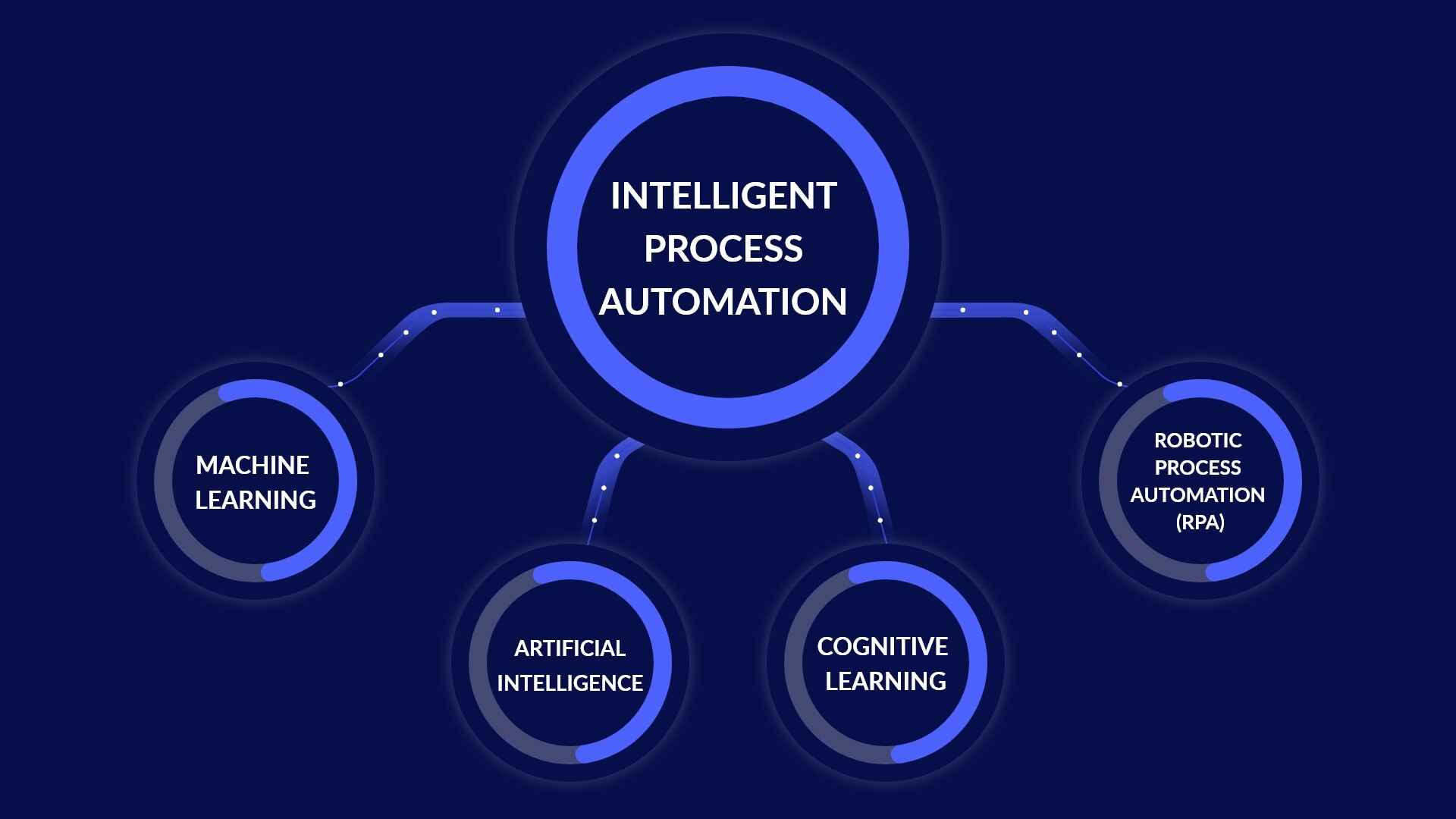
1. How Machine Learning Powers Automation
At the core of machine learning in business automation are algorithms capable of analyzing massive datasets, uncovering trends, and making accurate predictions. These models can classify information, detect anomalies, and make decisions with minimal human intervention. Unlike traditional software, ML models adapt and improve over time through continuous learning—making them ideal for dynamic business environments.
Businesses use ML techniques such as:
- Supervised learning, which involves training the model with labeled data for specific outputs (e.g., spam detection).
- Unsupervised learning, where the model finds hidden patterns in data without prior labeling (e.g., customer segmentation).
- Reinforcement learning, which allows algorithms to learn optimal actions through trial and error, often used in robotics or logistics.
This adaptability allows companies to automate everything from marketing campaigns to warehouse operations. As a result, businesses can reduce reliance on manual effort, enhance accuracy, and increase speed in delivering services.
2. Key Components of Machine Learning Automation
Implementing machine learning solutions involves a blend of software tools, infrastructure, and data science techniques. The following components are critical to powering ML-based automation:
- ML Frameworks: Open-source frameworks like TensorFlow, PyTorch, and Scikit-learn provide the foundation for building, training, and deploying ML models. These platforms offer modular tools for neural networks, natural language processing, and computer vision.
- Cloud-Based Services: Leading cloud providers—Amazon Web Services (AWS), Google Cloud Platform (GCP), and Microsoft Azure—offer ML-as-a-Service (MLaaS) solutions. These services allow businesses to scale quickly, process real-time data, and deploy predictive models globally.
- Data Pipelines: Robust data pipelines ensure seamless data collection, cleaning, transformation, and analysis. Tools like Apache Spark, Airflow, and Kubernetes are used to manage big data workflows.
- Model Monitoring and Maintenance: Post-deployment monitoring is crucial. ML models must be retrained periodically to maintain performance. Tools like MLflow and Kubeflow help in model tracking and lifecycle management.
Together, these components form a technology stack that enables efficient, scalable, and secure automation solutions.
3. Business Benefits of ML Solutions
Adopting machine learning automation unlocks a wide range of business benefits, including:
- Increased Operational Efficiency: ML models can process data at scale and speed far beyond human capabilities. This results in faster decision-making and reduced turnaround times.
- Cost Reduction: Automation of repetitive tasks lowers labor costs and reduces the need for manual intervention in time-consuming processes such as data entry, fraud detection, or document verification.
- Enhanced Customer Experience: ML-powered chatbots, recommendation engines, and sentiment analysis tools offer personalized experiences to customers, driving loyalty and satisfaction.
- Improved Risk Management: In sectors like banking or insurance, ML algorithms can detect fraudulent transactions, assess credit risk, and predict defaults with greater accuracy.
- Innovation and Competitive Edge: Companies that invest early in ML solutions often gain a strategic advantage, leveraging real-time insights to innovate products, optimize pricing, or anticipate market changes.
Whether it’s optimizing marketing strategies or enhancing product quality control, the implementation of custom ML solutions helps organizations align technology with business goals.
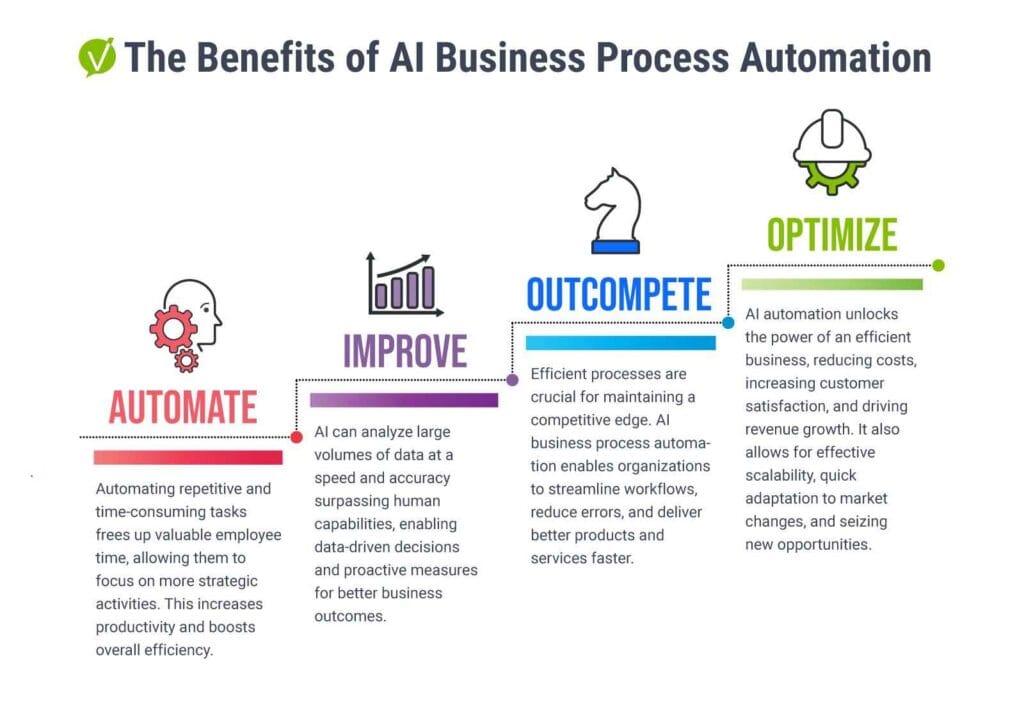
4. Real-World Use Cases Across Industries
Machine learning isn’t just a buzzword—it’s already delivering tangible value across multiple sectors:
Logistics and Supply Chain: ML improves route optimization, demand forecasting, and warehouse automation—streamlining the end-to-end logistics process.
Healthcare: ML helps in disease prediction, drug discovery, and personalized treatment plans. Algorithms analyze medical images, lab reports, and patient records to support faster and more accurate diagnoses.
Retail and E-commerce: Businesses use ML for inventory forecasting, dynamic pricing, and personalized product recommendations. ML-driven chatbots also enhance customer service by providing 24/7 support.
Finance and Banking: Fraud detection, algorithmic trading, and credit scoring are increasingly driven by machine learning models trained on financial data.
Manufacturing: Predictive maintenance, quality inspection, and production optimization are powered by ML and computer vision tools, reducing downtime and increasing efficiency.
Logistics and Supply Chain: ML improves route optimization, demand forecasting, and warehouse automation—streamlining the end-to-end logistics process.
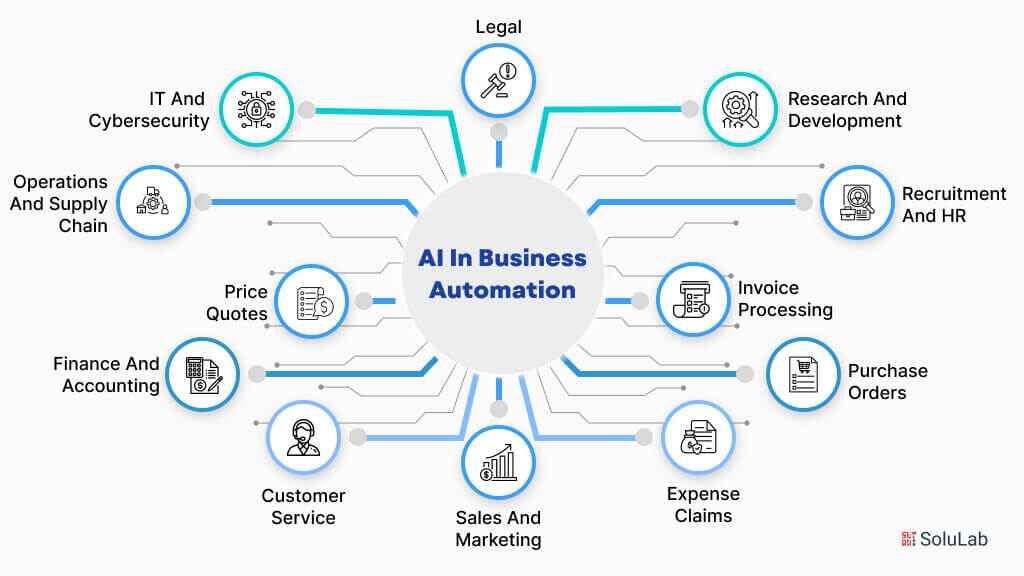
4. Real-World Use Cases Across Industries
Despite its advantages, adopting machine learning in business automation presents certain challenges:
- Data Privacy and Security: Businesses must comply with data regulations like GDPR and ensure that sensitive data is handled securely throughout the ML pipeline.
- Bias in Algorithms: If training data contains bias, ML models can perpetuate or amplify those biases. Organizations must prioritize fairness, accountability, and transparency.
- Skill Gaps: Building and managing ML systems requires expertise in data science, machine learning, and cloud architecture—skills that are still in high demand.
- Integration with Legacy Systems: Many enterprises operate on outdated infrastructure that may not support advanced ML solutions without significant upgrades.
- Model Interpretability: Some ML models, especially deep learning networks, are often perceived as “black boxes.” For high-stakes decisions, explainability and transparency are critical.
Overcoming these challenges involves adopting responsible AI practices, upskilling staff, and partnering with experienced ML vendors or consultants.
6. The Future of ML for Business
The role of machine learning in business automation is only set to expand. As technologies such as Edge Computing, Internet of Things (IoT), and Generative AI mature, ML will become more deeply integrated into every layer of enterprise operations.
Future developments to watch for include:
- Hyperautomation: Combining ML with robotic process automation (RPA) and AI to automate end-to-end business workflows.
- Autonomous Systems: From self-driving logistics fleets to autonomous financial trading bots, ML is powering systems that require minimal human intervention.
- AI-Powered Business Intelligence: Advanced analytics tools will deliver predictive insights that are instantly actionable, reshaping strategy and execution.
- Personalized AI Assistants: Virtual assistants tailored to individual employees or customers will handle more complex interactions and queries.
Companies that invest now in scalable and ethical ML infrastructure will be well-positioned to lead in their respective industries.
7. Conclusion
Machine learning is transforming the landscape of business automation, offering unprecedented opportunities to increase efficiency, cut costs, and deliver superior customer experiences. As ML algorithms become more sophisticated and accessible, even small and mid-sized businesses can leverage their power for growth and innovation.
By embracing ML automation, organizations can unlock new levels of productivity, tap into the full potential of their data, and future-proof their operations in an AI-driven world.
To stay updated on the latest advancements in machine learning, artificial intelligence, and digital transformation, visit www.updatevalley.com.